A tremendous amount of the work we do at DesignMap is human-to-human. We engage with stakeholders to build alignment, interview users to uncover their challenges, and provide design direction that propels organizations forward. All of these activities are deeply rooted in human interaction and context, which can make it a bit challenging to integrate Artificial Intelligence into our projects in a meaningful way.
But, there is one area where we believe AI could enhance the quality of our work: research (specifically, research synthesis). So, like anything else we do here at DesignMap, we decided to prototype, document, test, and refine our experience with these tools.
Over the next few months, we’ll share various perspectives on Designing with AI, including the benefits and drawbacks. For now, let's dive into using AI for research synthesis.
A couple preliminary notes:
- Our current goal in incorporating AI is not to increase efficiency, but to identify areas where AI can complement our work.
- While there are AI tools specifically designed for research, we will focus on general-purpose large language models (LLMs) like ChatGPT and Claude.
Context
In our initial experiment, we did a series of client interviews and synthesized the data using two different methods:
- Traditional process: A team analyzed interview notes and transcripts by breaking them down into key points on sticky notes, which were then clustered into themes across participants.
- AI-Assisted process: Another team used ChatGPT (GPT-4) to analyze the same transcripts and identify cross-cutting themes through a series of prompts.
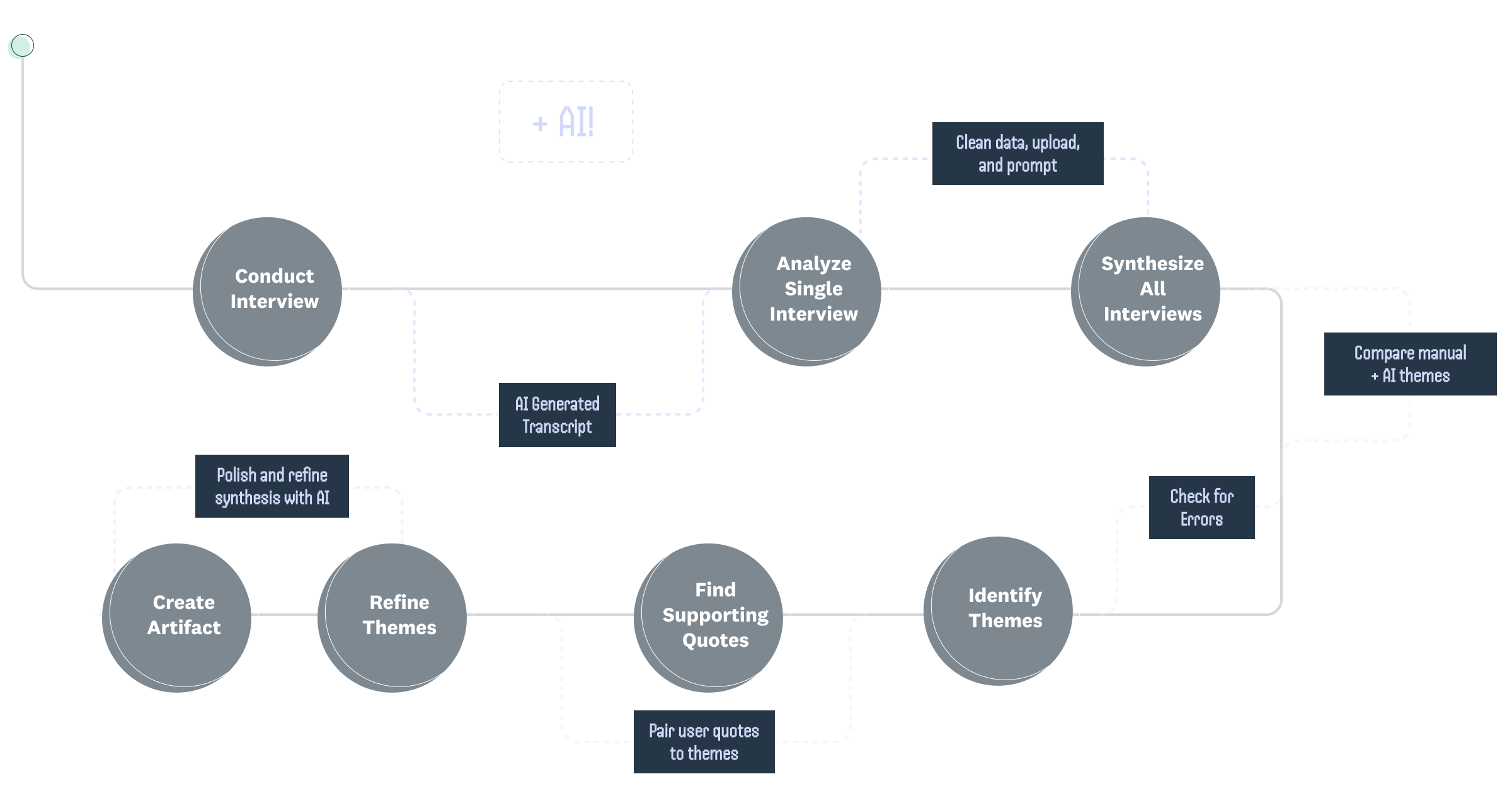
Here are three problems, three opportunities, and five tips we discovered when using general-purpose AI for research synthesis.
Three Problems with Using AI in Research Synthesis
- AI lacks EQ: Much of what is learned in interviews comes from non-verbal cues—speech cadence, tone, body language, facial expressions, pauses, and repetition. AI can miss these intangible, “softer” insights, which are crucial for understanding the human element of research. Flattening participants to interview text strips their humanity and makes it harder for stakeholders to empathize with their point of view.
- Identified themes were…basic: Generally, human-led synthesis produced more nuanced themes compared to those identified by GPT-4, which tended to be more straightforward. While AI can assist in identifying themes, it's best used after conducting your own synthesis. AI-generated themes can lead to confirmation bias, where you might seek evidence to support predefined ideas rather than exploring the raw data with an open mind. Combining human and AI synthesis ensures you don't overlook table stakes insights.
- Actually ADDS synthesis time: Using AI for research synthesis isn't a silver bullet. It requires significant manual work to prepare the data and provide accurate context for the AI tool. Errors in transcription (such as who is speaking) and context misinterpretation can lead to inaccurate conclusions. “Junk in, junk out,” as they say. Based on our experience, integrating AI into your research synthesis should be considered an additional track of work that demands careful time and effort management.
Three Opportunities with Using AI in Research Synthesis
- Find evidence faster: Identifying themes manually is time-consuming, but once you have themes, using a LLM to find supporting quotes can save considerable time. Reminder: as we mentioned above, teams should still familiarize themselves with the content to avoid unintentionally basing insights on incorrect or misunderstood data. In one instance, we saw AI only analyze insights from one interviewee and had to instruct it to include all participants for a more accurate, holistic picture.
- Discover overlooked insights: AI can highlight insights that might be missed in manual synthesis. Often, these insights are basic but valuable. For instance, ChatGPT identified that several participants worked at companies of similar size—an observation that might not have been explicitly noted in manual synthesis (but could inform a new avenue of exploration).
- Improve clarity and succinctness: AI excels at articulating themes and insights clearly and succinctly. While it might not always generate the right themes, using AI to refine and present your human-led findings can enhance clarity and make your synthesis more engaging.
Five Tips for Effectively Using AI in Research Synthesis
- Focus on efficacy over efficiency: It’s tempting to lead with AI synthesis in an effort to speed things up, but it’s important to prioritize firsthand observations (yes, that means listening to hours of recorded interviews!). This approach helps avoid AI errors and ensures a deeper, more accurate understanding of the research findings.
- Use AI as a supplement: Avoid starting your analysis with AI-generated themes. Doing so can constrain your critical thinking and lead to oversimplified conclusions. Instead, use AI to enhance and validate your own synthesis.
- Set AI up for success: To get the best results, provide detailed context about the research intent, background, and interviewees. Experiment with different instructions or even ask AI to adopt a certain persona to see how it affects the analysis.
- Clean your data: Quality input leads to quality output. Spend time cleaning up transcripts to correct mis-translations and remove filler words. Quick note about removing filler words: We tried to do this using ChatGPT, and it was awful! In some instances it substantially changed the words spoken. So, as always, check your AI tool’s work.
- Keep experimenting: The only way to learn is to keep experimenting. Try using AI for different research tasks: build your research protocol or experiment with telling AI to take on a persona to run research on.
While these insights are based on experiments with ChatGPT-4, we know there are several specialized AI research tools available, such as Monterey, InsightLab, Notably, CoNote, and Reveal. For anyone who has experience with these research-specific AI tools: we’d love to hear how/if they address some of the challenges we expressed.
Next up on our AI experiment list is using AI for more upstream research activities (like putting together a script or protocol).
Reach out to us (Will and/or Pooja) if you have experience with this—we’d love to hear more!